Modelling Behavior
Causal discovery to link neuronal circuits to behavior and plasticity
Classical statistical methods can uncover associations between neural activity patterns and behaviour but are unable to detect causal relations that would explain how neural activity controls behaviour.
In this project, we will build upon recent advances in machine learning on causal inference from observational data to develop method that are able to distinguish between correlation and causation to study how distributed activations of neuronal networks generate and support behaviour.
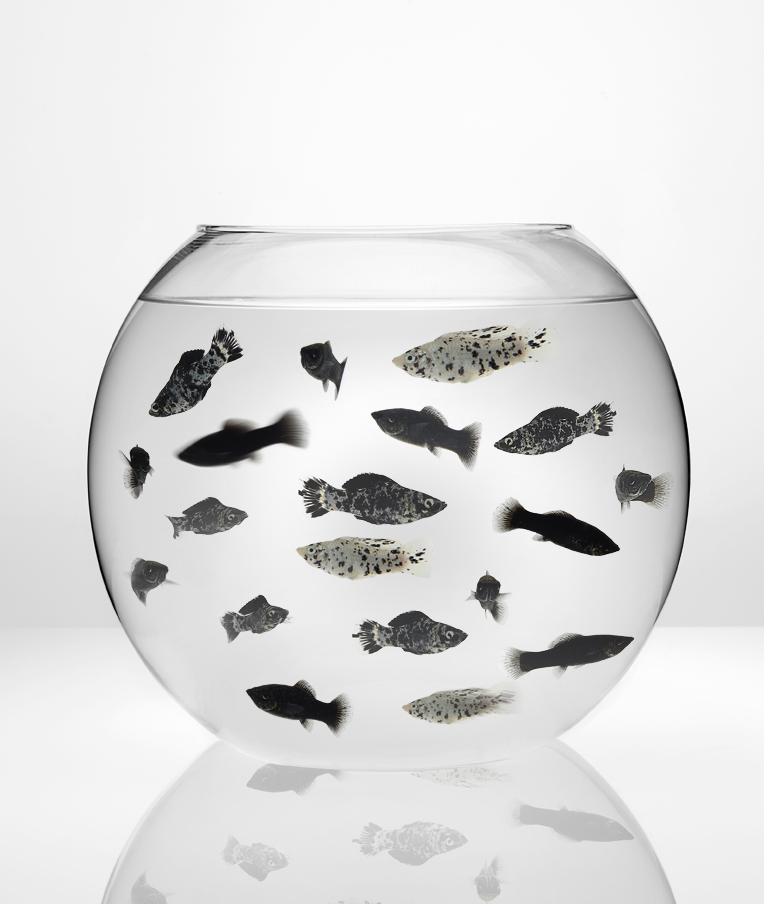
Publications
- P.K. Rubenstein, S. Weichwald, S. Bongers, J.M. Mooij, D. Janzing, M. Grosse-Wentrup, and B. Schölkopf [2017]. Causal consistency of structural equation models. In Proceedings of the Thirty-Third Annual Conference on Uncertainty in Artificial Intelligence (UAI 2017), page ID11.
- S. Weichwald, T. Meyer, O. Özdenizci, B. Schölkopf, T. Ball, and M. Grosse-Wentrup [2016]. Identification of causal relations in neuroimaging data with latent confounders: An instrumental variable approach. NeuroImage, 110:48–59.
- S. Weichwald, T. Meyer, O. Özdenizci, B. Schölkopf, T. Ball, and M. Grosse-Wentrup [2015]. Causal interpretation rules for encoding and decoding models in neuroimaging. NeuroImage, 110:48–59.